The Significance of Business in Machine Learning
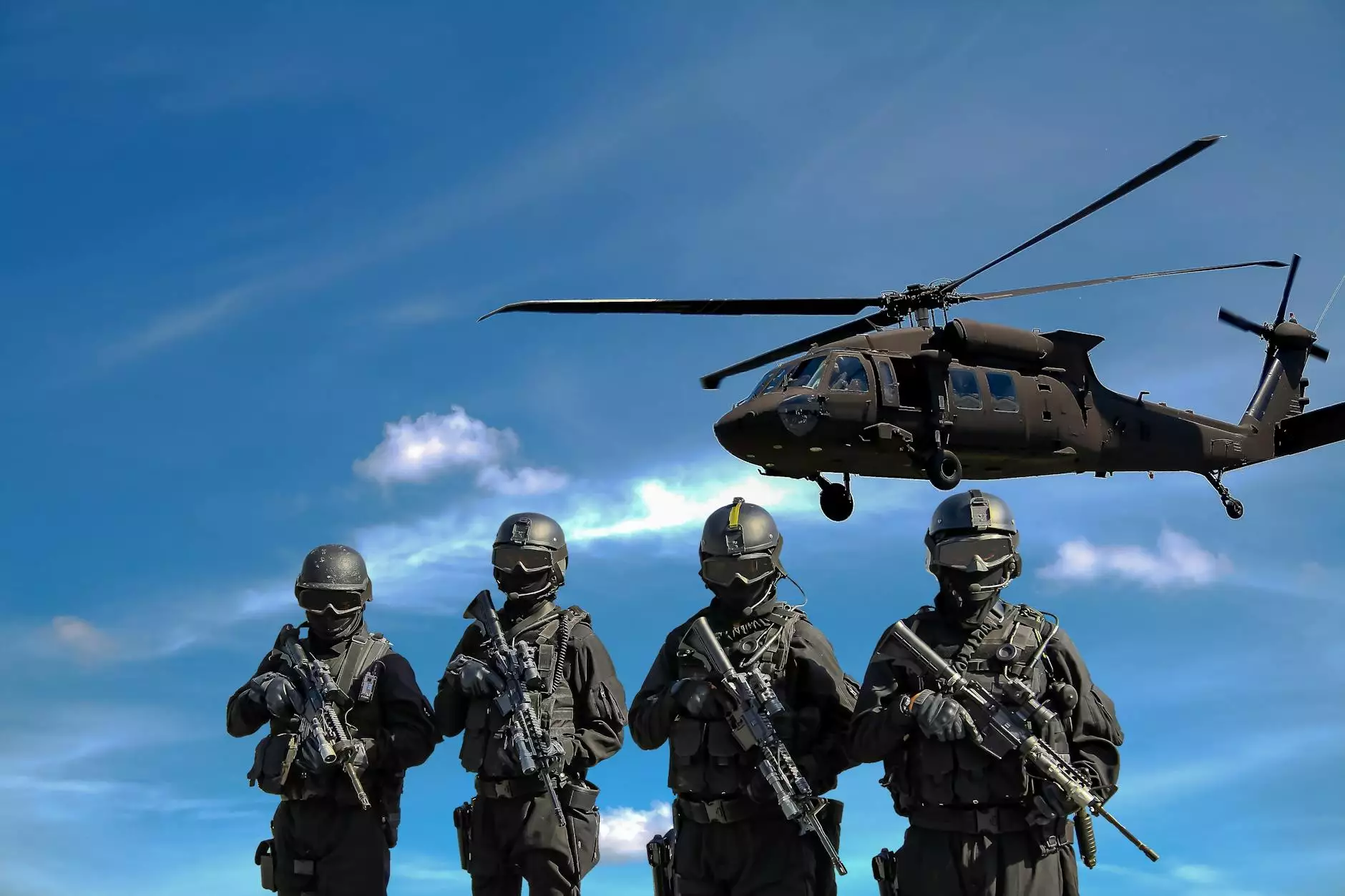
Machine learning (ML) has emerged as a transformative force across various sectors, fundamentally altering the landscape of business operations and strategic decision-making. In this article, we delve into the intersection of business and machine learning, drawing insights from the Journal of Machine Learning Research. Our aim is to provide a comprehensive overview of how businesses can leverage machine learning technologies to enhance efficiency, innovate processes, and improve customer experiences.
Understanding Machine Learning in Business Context
Machine learning refers to the subset of artificial intelligence that involves algorithms and statistical models that enable computers to perform tasks without explicit instructions. In a business context, ML plays a crucial role in data analysis, automation, and predictive analytics.
Key Applications of Machine Learning in Business
Businesses are increasingly adopting machine learning technologies to solve complex problems. Here are some key applications:
- Predictive Analytics: Using ML algorithms to analyze historical data and forecast future trends. This enables businesses to make informed decisions.
- Customer Segmentation: Identifying distinct customer groups based on purchasing behavior to tailor marketing efforts effectively.
- Fraud Detection: Developing algorithms that detect unusual patterns and behaviors to identify potential fraud in real-time.
- Supply Chain Optimization: Utilizing ML for demand forecasting, inventory management, and route optimization to streamline operations.
- Personalized Recommendations: Enhancing user experiences through personalized product recommendations based on user data.
The Role of the Journal of Machine Learning Research
The Journal of Machine Learning Research (JMLR) has been pivotal in disseminating knowledge and advancements in the field of machine learning. Its publications contain a wealth of information crucial for both academics and industry professionals.
Innovations Documented in the JMLR
Several noteworthy innovations highlighted in the JMLR have had profound implications for business applications:
- Deep Learning Models: Advances in neural networks, particularly deep learning, have opened new avenues for data interpretation and modeling complex datasets.
- Reinforcement Learning: Techniques that enable systems to learn optimal strategies over time through trial and error have been successfully implemented in various business scenarios.
- Transfer Learning: Approaches that allow knowledge gained in one domain to be applied in another, drastically reducing the amount of data required for training models.
Challenges and Considerations in Implementing Machine Learning
While machine learning presents numerous opportunities, businesses also face several challenges:
Data Quality and Availability
The effectiveness of machine learning algorithms significantly depends on the quality and quantity of data available. Businesses must ensure that the data they collect is clean, relevant, and accessible. Moreover, organizations must address issues of data privacy and compliance, especially when dealing with sensitive customer information.
Understanding Algorithms
Many business leaders may lack the technical expertise to understand how ML algorithms work. It's crucial for organizations to foster collaboration between data scientists and business stakeholders to bridge this gap.
Integration into Business Processes
Integrating machine learning solutions into existing workflows can be complex. Businesses need to allocate resources effectively and ensure that there is a clear strategy for implementation.
Strategies for Successful Machine Learning Adoption in Business
To successfully harness the potential of machine learning, businesses should consider the following strategies:
1. Start Small, Scale Gradually
Begin with pilot projects that address specific business needs. After initial successes, scale up gradually to minimize risks and ensure sustainable growth.
2. Invest in Infrastructure
Robust IT infrastructure is essential for supporting machine learning initiatives. Implementing cloud-based solutions can offer the necessary scalability and flexibility.
3. Cultivate a Data-Driven Culture
Encourage a culture that values data-driven decision-making. Educating staff about the benefits and workings of machine learning can foster acceptance and innovation.
4. Collaborate with Experts
Partnering with academic institutions, researchers, or consultants can provide additional expertise and insights, facilitating more effective implementation strategies.
The Future of Business and Machine Learning
As we look towards the future, the relationship between business and machine learning is bound to deepen. The evolution of ML technologies will unlock new potential, fostering innovation and creating more efficient workflows. Among the trends that are shaping the future, we can highlight:
- Automation of Routine Tasks: Increasing automation will allow businesses to focus on strategic tasks while reducing operational costs.
- Enhanced Customer Insights: Analyzing customer data will enable businesses to develop tailored products and services, improving customer satisfaction.
- Ethical AI Development: As companies adopt ML, the emphasis on ethical considerations and transparency in machine learning algorithms will become paramount.
Conclusion
In conclusion, the synergy between business and machine learning is not just a trend—it's a necessity in the modern marketplace. Companies that acknowledge and adapt to the changes brought about by machine learning, as discussed in the Journal of Machine Learning Research, will undoubtedly have a competitive advantage. As we move forward, embracing these technologies responsibly and effectively will define the leaders of the future. Organizations must remain proactive in their strategies, ensuring that they are not left behind in this rapidly evolving landscape.