Unlocking the Power of Quality Image Annotation for Business Success
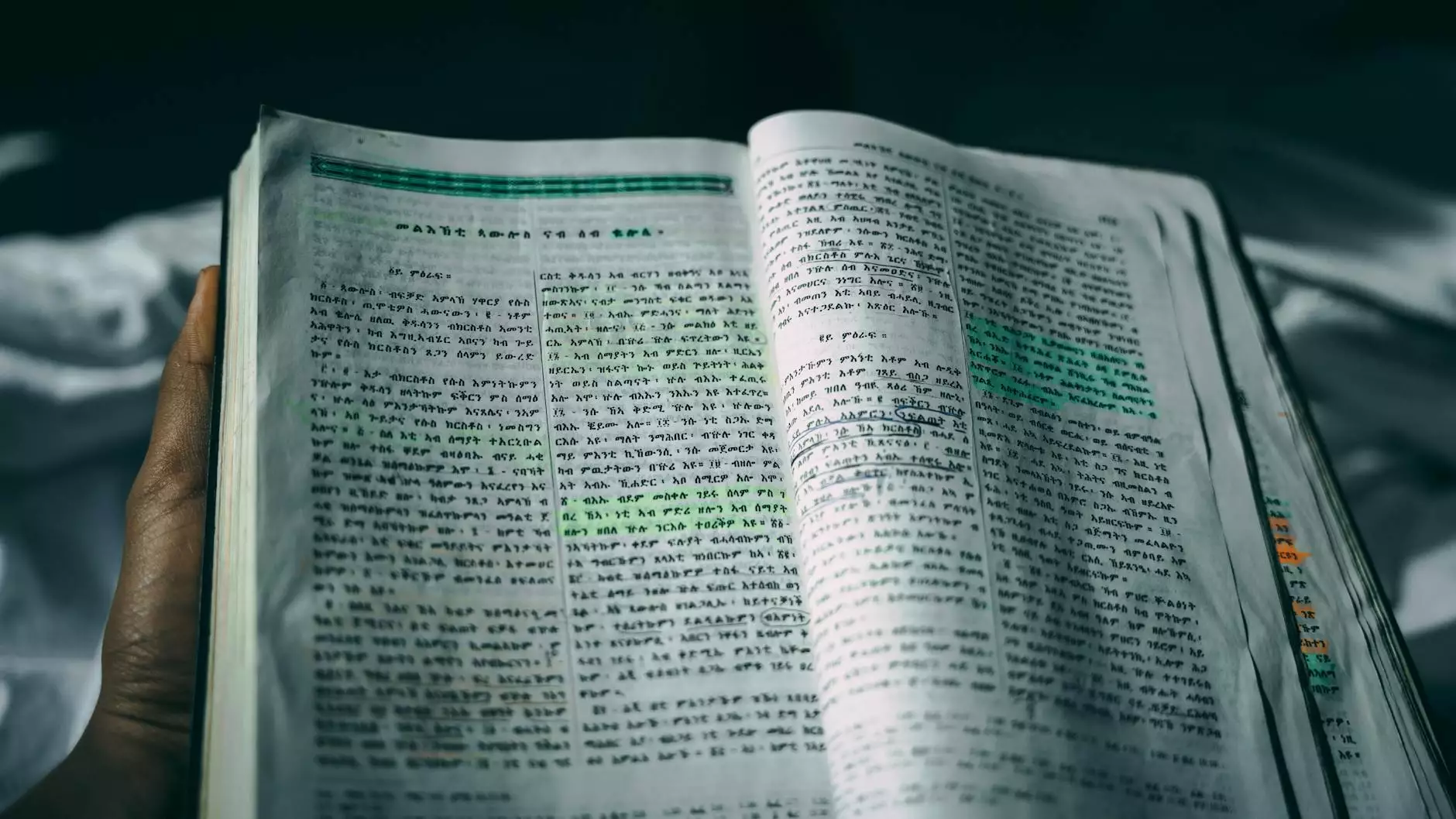
The digital landscape is rapidly evolving, and businesses are increasingly relying on data-driven strategies to remain competitive. At the heart of this transformation lies the significance of quality image annotation, a crucial process that enhances machine learning models, empowers AI algorithms, and ultimately drives intelligent decision-making. In this article, we delve into the intricacies of quality image annotation, its benefits, and how platforms like Keylabs.ai are redefining the standards for data annotation tools and platforms.
Understanding Quality Image Annotation
Quality image annotation refers to the meticulous process of labeling images so that machines can understand and interpret the visual data. This is more than just adding tags to images; it involves a variety of techniques tailored to the specific needs of businesses and their data. The effectiveness of AI and machine learning models heavily relies on the quality of the annotated data they are fed. Thus, achieving precision and accuracy in image annotation is paramount.
Types of Image Annotation
There are several methods of image annotation, each serving different purposes in various fields. Here are some of the most common types:
- Bounding Boxes: Defining rectangles around objects to identify them.
- Semantic Segmentation: Assigning a class to each pixel in an image, often used in autonomous driving.
- Instance Segmentation: Similar to semantic segmentation, but differentiates between overlapping objects.
- Landmark Annotation: Marking specific points or landmarks on an image, significant in facial recognition.
- Polygon Annotation: Using polygons to outline complex shapes in images.
The Importance of Quality in Image Annotation
With the surge of data being generated, the importance of quality in image annotation cannot be overstated. Here are several key reasons why quality matters:
1. Enhanced Model Performance
The performance of machine learning models is directly proportional to the quality of annotated data. High-quality annotations ensure that the model learns effectively, reduces errors, and improves prediction accuracy. Businesses aiming for high-performance AI models will find that investing in quality image annotation pays off significantly.
2. Increased Efficiency
Quality image annotation streamlines workflows and reduces the time spent on data cleaning and correction. When data is accurately labeled from the beginning, teams can focus on developing algorithms rather than fixing errors, leading to improved project timelines and cost savings.
3. Boosted Trust in AI Decisions
As AI systems become integrated into critical business processes, stakeholders need to trust the decisions made by these systems. Quality image annotation contributes to building this trust by providing reliable data that underlies AI predictions, thereby ensuring that outcomes are justified and accurate.
4. Scalability of AI Solutions
Businesses are often faced with large volumes of image data. Quality image annotation enables scalable solutions that can handle significant datasets efficiently. This is particularly important for industries that rely on continuous data input, such as healthcare and automotive sectors.
Choosing the Right Data Annotation Platform
When it comes to implementing quality image annotation, the choice of data annotation platform can make all the difference. Here’s what to look for:
1. User-Friendly Interface
A platform with an intuitive interface allows annotators to work more efficiently. The faster they can annotate, the more productive the process becomes.
2. Robust Features
Look for platforms that offer comprehensive features for different types of annotation, such as the capabilities within Keylabs.ai. They should support multiple annotation types such as bounding boxes, segmentation, and more.
3. Quality Assurance Processes
Ensure your chosen annotation tool has built-in quality assurance processes to maintain high standards throughout the annotation workflow.
4. Scalability
A good annotation platform should be able to scale with your business needs, accommodating larger datasets and more complex projects as they arise.
5. Collaboration Features
Collaboration tools enable teams to work together more efficiently, facilitating communication between annotators and project managers for better results.
Implementing Quality Image Annotation in Your Business
To effectively integrate quality image annotation into your business operations, consider the following steps:
1. Define Objectives
Understand what you aim to achieve with image annotation. Are you developing a computer vision application? Are you seeking to enhance your product offerings with AI? Define clear objectives to inform your annotation strategy.
2. Choose the Right Tools
Select a data annotation tool that aligns with your requirements. Platforms like Keylabs.ai can provide tailored solutions to meet your annotation needs efficiently.
3. Train Your Team
Invest in training your team on the importance of quality image annotation. Regular workshops and updates on best practices can help maintain high standards across your annotation projects.
4. Monitor and Adjust
Continuous monitoring of the annotation process is crucial. Collect feedback from annotators and machine learning teams to identify areas for improvement.
Future Trends in Image Annotation
The field of image annotation is continuously evolving, driven by advancements in technology and changing industry needs. Here are some trends to watch:
1. Automation and AI Assistance
As machine learning models become more sophisticated, there will be an increase in automation in the annotation process. AI-assisted annotation tools will help annotators work faster while maintaining quality.
2. Integration with AI Models
Future platforms will likely offer deeper integration with AI models, providing feedback loops that improve annotations based on model performance.
3. Collaborative Annotation Platforms
The need for collaborative tools will grow, allowing teams from different geographical locations to work together seamlessly on annotation tasks.
4. Enhanced Quality Assurance Techniques
Implementing more sophisticated QA techniques, such as machine learning algorithms for error detection, will become common to maintain high annotation quality.
Conclusion: Quality Image Annotation as a Strategic Asset
In a world increasingly dominated by data, the significance of quality image annotation cannot be underestimated. It serves as a backbone for machine learning and AI applications, enabling businesses to harness actionable insights and drive innovation. By selecting the right tools and adhering to best practices, companies can position themselves for success in their respective industries. Keylabs.ai is at the forefront of providing exceptional data annotation tools and platforms that cater to the evolving needs of modern businesses. Embrace quality image annotation as a strategic asset and unlock your business's full potential.