Maximizing Efficiency with Labeling Tools for Machine Learning
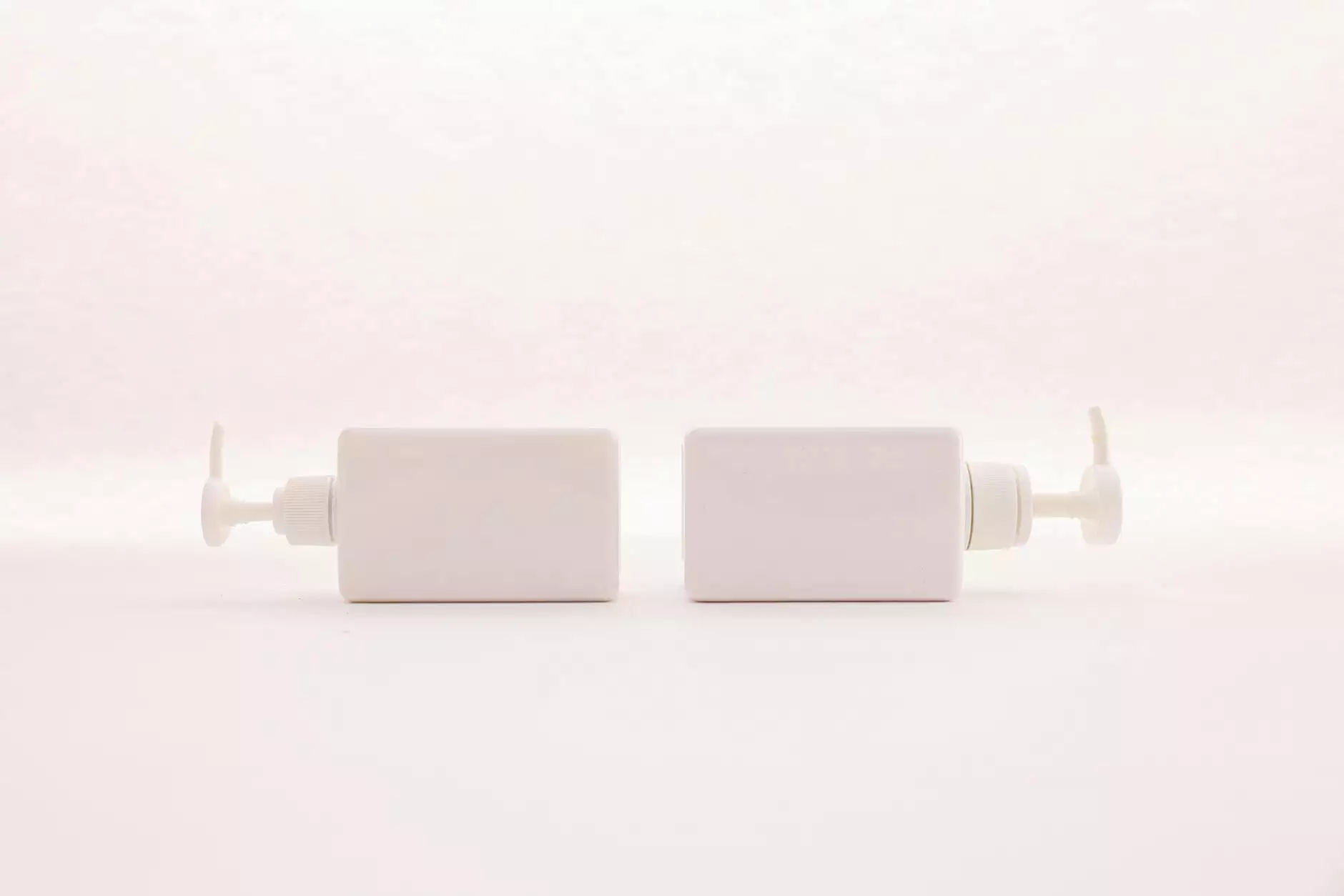
In the rapidly evolving landscape of *machine learning*, the efficiency of data preparation can greatly impact the success of projects. One of the crucial components in this preparation stage is utilizing effective labeling tools for machine learning. Whether you're a budding entrepreneur or an established business, understanding how these tools work can significantly enhance your productivity and output quality.
Understanding the Importance of Data Labeling
Data labeling is the process of tagging or annotating data, so machines can understand and learn from it. This practice is essential for training machine learning algorithms, allowing them to recognize patterns and make predictions based on that data. Without properly labeled data, even the most advanced algorithms can fail, leading to poor results and inefficiencies.
Types of Data that Require Labeling
Different types of data benefit from specific labeling strategies:
- Image Data: Labeling involves identifying objects within images, such as car models, faces, or various items.
- Text Data: Assigning tags to words or phrases to determine sentiment, intent, or context.
- Audio Data: Transcribing or categorizing sounds or speech for tasks like voice recognition.
- Video Data: Annotating video segments with labels for actions, people, or objects.
Benefits of Using Labeling Tools for Machine Learning
Businesses that incorporate effective labeling tools into their machine learning processes can reap significant rewards.
1. Enhanced Accuracy and Consistency
Labeling tools can greatly improve the accuracy of labeled data. By using predefined guidelines and templates, these tools ensure consistent labeling across datasets. This reduces the risk of human error, which can often lead to misleading outcomes.
2. Increased Efficiency
Automating parts of the labeling process saves time and resources. Businesses can focus more on analysis and model development rather than spending excessive time on data preparation. This efficiency can lead to quicker project turnaround times.
3. Scalability
As your data needs grow, so does the challenge of labeling. Using advanced labeling tools allows businesses to scale their operations without significantly increasing costs or manpower. This flexibility ensures that companies can meet growing demands without sacrificing quality.
4. Improved Collaboration
Many labeling tools provide features that streamline collaboration among teams. With cloud-based platforms, teams can work simultaneously on projects, share insights, and manage data more effectively. This collaboration fosters innovation and quick problem-solving.
Choosing the Right Labeling Tools for Your Business
With various options available, selecting the right labeling tool for your needs is critical. Consider the following factors:
1. Functionality and Features
The first step in choosing a labeling tool is assessing its functionality. Look for tools that offer the specific features you need, such as:
- User-friendly interfaces
- Support for multiple data types
- Integration capabilities with your existing systems
- Collaboration tools for team projects
2. Scalability and Flexibility
Your chosen tool should easily adapt as your data labeling demands grow. Ensure that the vendor can accommodate increases in volume and complexity without decreasing performance.
3. Cost-Effectiveness
While investing in high-quality tools is essential, businesses must also evaluate the cost relative to their budgets. Compare different tools to find a solution that offers the best value without compromising quality.
Top Labeling Tools for Machine Learning in 2023
Here’s a list of some of the most effective labeling tools that have proven invaluable for machine learning projects:
- Labelbox: A feature-rich platform suitable for various data types, offering user-friendly interfaces and integration capabilities.
- Amazon SageMaker Ground Truth: This tool provides an efficient way to create highly accurate training datasets through human labeling and machine learning.
- SuperAnnotate: Designed for teams, it facilitates collaboration and supports numerous datasets, making it ideal for large projects.
- DataRobot: Ideal for businesses looking for an all-in-one solution that not only labels data but also optimizes machine learning workflows.
- VGG Image Annotator: A free tool specifically for image data, allowing users to annotate images quickly and efficiently.
Implementing Labeling Tools with Your Workflow
Integrating labeling tools into your machine learning workflow requires strategic planning. Follow these steps for a smooth implementation:
1. Assess Your Data Needs
Before implementing any tool, understand your data requirements. What type of data are you dealing with? What volume do you anticipate? Clarifying these points will guide your tool selection.
2. Train Your Team
A tool is only as good as the people using it. Ensure that your team is well-trained on how to utilize the labeling tools effectively. Offer workshops or online resources for continuous learning.
3. Monitor Performance and Accuracy
Once implemented, consistently monitor the performance of your labeling process. Regular audits will help ensure that your labeled data maintains high quality, which is essential for successful machine learning outcomes.
Common Challenges in Data Labeling
While utilizing labeling tools can streamline processes, several challenges persist:
1. Data Privacy Concerns
With the handling of sensitive data, ensuring privacy is crucial. Businesses must choose tools that comply with data protection regulations and best practices.
2. Addressing Labeling Bias
Labeling bias can occur when data is labeled inconsistently or inaccurately. This can lead to skewed machine learning models. Continuous evaluation and diverse labeling teams can help mitigate this risk.
3. Keeping Pace with Evolving Needs
As technology advances, so do the expectations for data labeling. Businesses must stay updated with industry trends to ensure their labeling processes remain effective and relevant.
Conclusion
In summary, labeling tools for machine learning represent an essential component in the data preparation phase of any machine learning project. By enhancing accuracy and efficiency, these tools empower businesses to achieve outstanding results. As you consider implementing such tools within your operations, remember to assess your specific needs, equip your team, and continuously monitor your processes. By doing so, you can leverage the full potential of machine learning to transform your business practices.
About KeyMakr
KeyMakr specializes in providing high-quality home services, particularly in the fields of keys and locksmiths. Our mission is to enhance security and efficiency for our clients while ensuring reliable service. By integrating advanced technologies and tools into our operations, we endeavor to stay ahead of the curve, providing the best services possible.